Cognitive Computing
Introduction
Cognitive computing refers to the simulation of human thought processes in a computerized model. This involves self-learning systems that utilize data mining, pattern recognition, and natural language processing to mimic the way a human brain works. The goal of cognitive computing is to create automated IT systems that are capable of solving problems without human intervention.
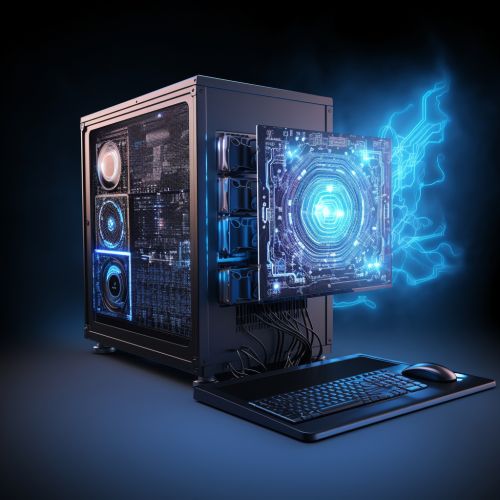
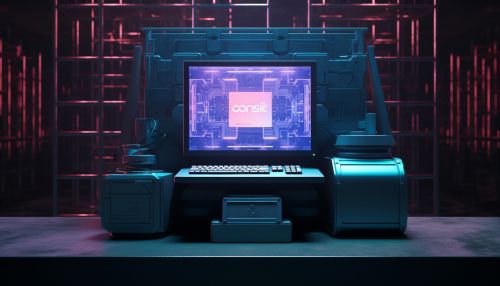
History and Evolution
The concept of cognitive computing originated from the field of artificial intelligence (AI). The term was first coined by IBM with the launch of their Watson supercomputer, which was designed to demonstrate the capabilities of cognitive computing. The evolution of cognitive computing has been driven by advancements in machine learning algorithms and improvements in computational hardware that have made it possible to process large amounts of data in real time.
Key Components of Cognitive Computing
Cognitive computing systems are composed of several key components, which include:
Machine Learning
Machine learning is a method of data analysis that automates the building of analytical models. It is a branch of artificial intelligence based on the idea that systems can learn from data, identify patterns and make decisions with minimal human intervention.
Natural Language Processing
Natural language processing (NLP) is a field of artificial intelligence that gives the machines the ability to read, understand, and derive meaning from human languages. It is a critical component of cognitive computing systems as it allows them to understand and respond to human language in a natural and intuitive way.
Data Mining
Data mining is the process of discovering patterns in large data sets involving methods at the intersection of machine learning, statistics, and database systems. It is an essential part of cognitive computing as it allows the system to learn from the data and make informed decisions.
Pattern Recognition
Pattern recognition is the automated recognition of patterns and regularities in data. It is a fundamental part of cognitive computing as it allows the system to recognize patterns in the data and make predictions based on these patterns.
Applications of Cognitive Computing
Cognitive computing has a wide range of applications across various sectors. Some of the most notable applications include:
Healthcare
In the healthcare sector, cognitive computing can be used to provide personalized treatment plans based on a patient's medical history and current health status. It can also be used to predict potential health risks and suggest preventive measures.
Finance
In the finance sector, cognitive computing can be used to analyze financial data and make predictions about future market trends. It can also be used to detect fraudulent transactions and improve risk management.
Retail
In the retail sector, cognitive computing can be used to analyze customer behavior and preferences to provide personalized shopping experiences. It can also be used to optimize inventory management and improve supply chain efficiency.
Education
In the education sector, cognitive computing can be used to provide personalized learning experiences based on a student's learning style and performance. It can also be used to develop intelligent tutoring systems that can adapt to the needs of individual students.
Challenges and Limitations
While cognitive computing holds great promise, it also presents several challenges and limitations. These include:
Data Privacy and Security
As cognitive computing systems rely on large amounts of data to function effectively, ensuring data privacy and security is a major challenge. There are concerns about the potential misuse of personal data and the risk of data breaches.
Ethical Considerations
There are also ethical considerations associated with cognitive computing. For instance, there are concerns about the potential for bias in decision-making processes and the impact of automation on employment.
Technical Challenges
There are also technical challenges associated with cognitive computing. These include the need for large amounts of computational power and the complexity of developing and maintaining cognitive computing systems.
Future of Cognitive Computing
The future of cognitive computing looks promising, with advancements in technology and increased adoption across various sectors. As cognitive computing systems continue to evolve, they are expected to become more sophisticated and capable of handling more complex tasks. This could lead to significant improvements in efficiency and productivity across various sectors.