Clinical Decision Support Systems
Introduction
Clinical Decision Support Systems (CDSS) are computer-based programs that assist healthcare professionals in making clinical decisions. These systems are designed to improve healthcare delivery by providing accurate, timely and relevant information to clinicians. CDSS use a variety of data to provide evidence-based recommendations, helping to enhance patient care and reduce medical errors.
History
The concept of CDSS emerged in the 1970s with the advent of more sophisticated computer technologies. Early systems were primarily rule-based, relying on if-then logic to generate advice. However, these systems were limited by the complexity of medical knowledge and the difficulty of translating this knowledge into rules. In the 1980s and 1990s, the development of artificial intelligence (AI) and machine learning techniques allowed for the creation of more advanced CDSS. These systems could learn from data and improve their performance over time, leading to more accurate and personalized recommendations.
Types of CDSS
There are two main types of CDSS: knowledge-based and non-knowledge-based systems.
Knowledge-based CDSS rely on a set of rules and relationships defined by experts. These systems use a problem-solving process to generate recommendations.
Non-knowledge-based CDSS, on the other hand, use artificial intelligence techniques, such as machine learning and neural networks, to learn from data and generate advice. These systems can handle more complex problems and provide more personalized recommendations.
Components of CDSS
A typical CDSS consists of three main components: a clinical knowledge base, an inference engine, and a communication mechanism.
The clinical knowledge base contains the medical knowledge necessary for decision making. This knowledge is usually represented as rules, algorithms, or pathways.
The inference engine is the part of the system that applies the knowledge to patient data to generate recommendations.
The communication mechanism is responsible for presenting the recommendations to the user in a clear and understandable way.
Benefits of CDSS
CDSS have several potential benefits. They can improve the quality of care by providing evidence-based recommendations, reducing variability in practice, and preventing medical errors. They can also improve efficiency by reducing the time needed for decision making and increasing the speed of care delivery. Furthermore, CDSS can enhance learning and knowledge retention among healthcare professionals by providing educational feedback.
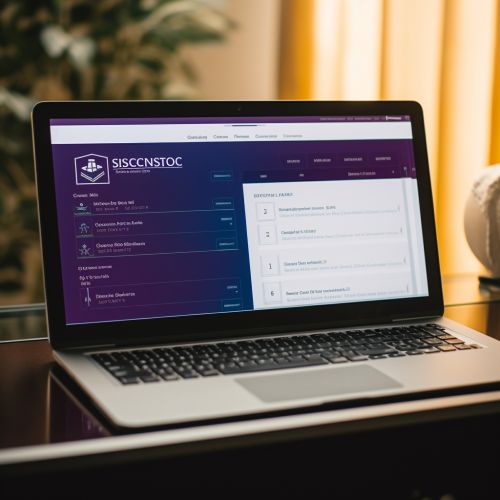
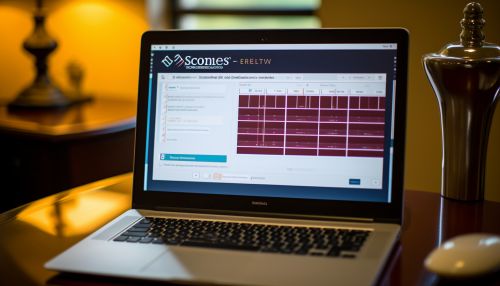
Challenges and Limitations
Despite their potential benefits, CDSS also have several challenges and limitations. These include the difficulty of maintaining and updating the clinical knowledge base, the risk of over-reliance on the system, and the potential for alert fatigue. Moreover, the effectiveness of CDSS can be influenced by factors such as the design of the system, the context of use, and the characteristics of the users.
Future Directions
The future of CDSS is likely to be influenced by advances in technology and changes in healthcare delivery. Potential future directions include the integration of CDSS with electronic health records, the use of big data and predictive analytics, and the development of patient-centered CDSS.