Clinical Decision Support System
Introduction
A Clinical Decision Support System (CDSS) is a sophisticated application designed to aid healthcare professionals in making clinical decisions. These systems integrate patient data with medical knowledge to generate case-specific advice, enhancing the quality of care and improving patient outcomes. CDSSs are integral to modern healthcare, providing support in diagnosis, treatment planning, and disease management. They operate by utilizing various forms of data, including electronic health records (EHRs), clinical guidelines, and patient-specific information, to deliver evidence-based recommendations.
Historical Development
The evolution of CDSS can be traced back to the 1960s, with early systems like MYCIN and INTERNIST-I pioneering the field. MYCIN, developed at Stanford University, was one of the first rule-based systems designed to diagnose bacterial infections and recommend antibiotics. Despite its innovative approach, MYCIN was never used clinically due to concerns about its reliability and the complexity of integrating it into existing workflows.
Over the decades, advancements in AI and computing power have significantly enhanced the capabilities of CDSSs. The integration of machine learning algorithms has enabled these systems to process vast amounts of data and learn from clinical outcomes, thereby improving their accuracy and reliability.
Components of CDSS
A typical CDSS comprises several key components:
Knowledge Base
The knowledge base is the core of any CDSS, containing the medical knowledge necessary for decision-making. This includes clinical guidelines, treatment protocols, and evidence-based research. The knowledge base can be static, relying on predefined rules, or dynamic, incorporating machine learning to update its content based on new data.
Inference Engine
The inference engine processes the information from the knowledge base and patient data to generate recommendations. It uses algorithms to match patient-specific data with the knowledge base, producing diagnostic or therapeutic suggestions. The inference engine can employ various techniques, such as rule-based reasoning, Bayesian networks, or neural networks.
Communication Mechanism
The communication mechanism is responsible for delivering the system's recommendations to the healthcare provider. This can be through alerts, reminders, or detailed reports. The effectiveness of a CDSS often depends on how well it integrates into the clinical workflow, ensuring that recommendations are timely and actionable.
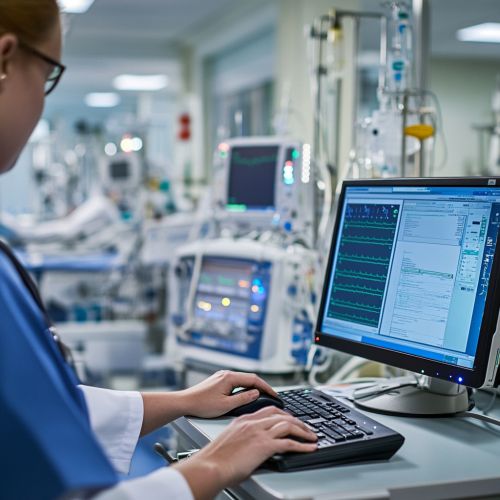

Types of CDSS
CDSSs can be categorized based on their functionality and the type of support they provide:
Diagnostic Support Systems
These systems assist clinicians in diagnosing diseases by analyzing patient data and comparing it with known disease patterns. They are particularly useful in complex cases where multiple conditions may present similar symptoms.
Therapeutic Support Systems
Therapeutic support systems provide guidance on treatment options, including drug prescriptions, dosage calculations, and potential interactions. They help ensure that treatments are tailored to individual patient needs and comply with the latest clinical guidelines.
Prognostic Support Systems
Prognostic systems predict patient outcomes based on current clinical data and historical trends. They are valuable in chronic disease management, helping clinicians anticipate complications and adjust treatment plans accordingly.
Implementation Challenges
Despite their potential benefits, implementing CDSSs in clinical practice poses several challenges:
Data Integration
Integrating CDSSs with existing EHR systems is often complex, requiring interoperability between different software platforms. Ensuring seamless data flow is crucial for the accuracy and reliability of the system's recommendations.
User Acceptance
The success of a CDSS depends on its acceptance by healthcare providers. Systems that are perceived as intrusive or difficult to use may face resistance from clinicians. Therefore, user-friendly interfaces and minimal workflow disruption are essential for widespread adoption.
Legal and Ethical Considerations
CDSSs raise important legal and ethical questions, particularly concerning liability and patient privacy. Determining responsibility for clinical decisions made with the aid of a CDSS is a complex issue, as is ensuring that patient data is protected in compliance with regulations like the Health Insurance Portability and Accountability Act (HIPAA).
Future Directions
The future of CDSSs lies in the continued integration of AI and big data analytics. As these technologies advance, CDSSs will become more personalized, offering recommendations tailored to individual genetic profiles and lifestyle factors. Additionally, the rise of telemedicine and remote monitoring will expand the reach of CDSSs, enabling real-time decision support for patients in diverse settings.