COCO dataset
Overview
The Common Objects in Context (COCO) dataset is a large-scale object detection, segmentation, and captioning dataset. It is a widely used resource in the field of computer vision, providing a diverse set of images with comprehensive annotations that allow for the training and evaluation of algorithms for a variety of tasks, such as object detection, instance segmentation, and image captioning.
History
The COCO dataset was first released in 2014 by a team of researchers from Microsoft. The goal was to address the need for a dataset that could be used to train models for object detection and segmentation tasks that are more complex than those addressed by existing datasets, such as ImageNet and Pascal VOC. The dataset has been updated several times since its initial release, with the most recent version being released in 2017.
Dataset Composition
The COCO dataset consists of 330,000 images, 200,000 of which are labeled. The images are collected from the internet and represent a wide variety of contexts, including urban and rural settings, indoor and outdoor scenes, and a range of weather and lighting conditions.
The dataset includes annotations for 80 object categories, which are grouped into 12 high-level categories: person, animal, vehicle, outdoor, accessory, sports, kitchen, food, furniture, electronic, appliance, and indoor. Each image in the dataset is annotated with bounding boxes for each object, as well as pixel-level segmentation masks for each object instance.
Annotation Process
The annotation process for the COCO dataset involves both automated and manual steps. First, object candidates are generated using a combination of Edge Boxes and Selective Search. These candidates are then manually annotated by human workers, who draw bounding boxes around each object and label it with its category.
For the instance segmentation task, the workers also draw a pixel-level mask for each object instance. This process is facilitated by a custom web-based tool, which allows the workers to draw polygons around each object and then fill in the interior of the polygon to create the mask.
Use in Research
The COCO dataset has been widely used in computer vision research, particularly in the areas of object detection and instance segmentation. It has been used to train and evaluate a variety of models, including Faster R-CNN, Mask R-CNN, and YOLO.
The dataset is also used in the annual COCO Challenge, a competition that encourages researchers to develop and test new algorithms for object detection, instance segmentation, and image captioning. The challenge provides a standardized benchmark for comparing the performance of different algorithms, and the results are presented at the Conference on Computer Vision and Pattern Recognition (CVPR).
Limitations
While the COCO dataset is a valuable resource for computer vision research, it is not without its limitations. One limitation is that the dataset is heavily biased towards images from the internet, which may not accurately represent the diversity of real-world scenes. Additionally, the dataset only includes 80 object categories, which is significantly fewer than the thousands of categories included in datasets like ImageNet.
Another limitation is the quality of the annotations. While the manual annotation process ensures a high level of accuracy, it is also time-consuming and expensive. As a result, the dataset includes a large number of unlabeled images, which limits its usefulness for supervised learning tasks.
See Also
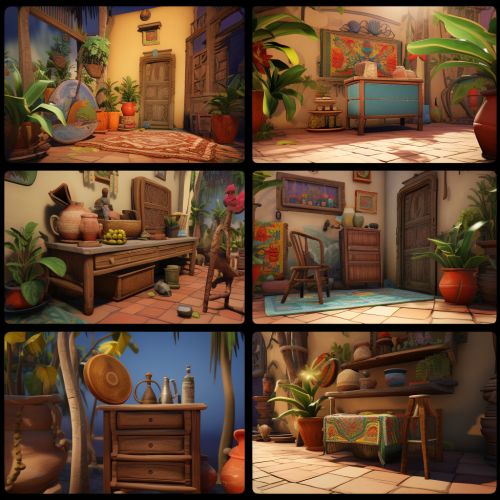
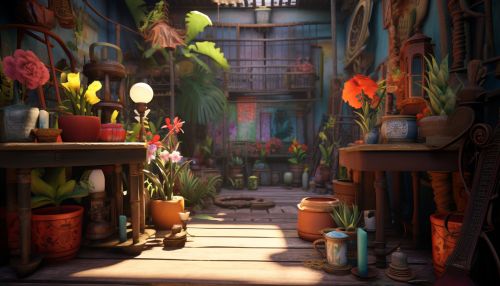