Data cleansing: Difference between revisions
(Created page with "== Introduction == Data cleansing, also known as data cleaning or data scrubbing, is the process of detecting and correcting (or removing) corrupt or inaccurate records from a dataset, table, or database. It involves identifying incomplete, incorrect, inaccurate, or irrelevant parts of the data and then replacing, modifying, or deleting this dirty data. Data cleansing is crucial for ensuring the quality and reliability of data used in various applications, including busi...") |
No edit summary |
||
Line 32: | Line 32: | ||
Data enrichment involves enhancing the quality of data by adding additional information from external sources. This can include appending missing information, correcting inaccuracies, or adding new attributes. For example, enriching customer data with demographic information can provide more insights for marketing analysis. | Data enrichment involves enhancing the quality of data by adding additional information from external sources. This can include appending missing information, correcting inaccuracies, or adding new attributes. For example, enriching customer data with demographic information can provide more insights for marketing analysis. | ||
[[Image:Detail-95959.jpg|thumb|center|A team of data scientists working on data cleansing, with computer screens displaying datasets and data quality metrics.]] | |||
== Tools and Technologies for Data Cleansing == | == Tools and Technologies for Data Cleansing == |
Revision as of 22:06, 3 July 2024
Introduction
Data cleansing, also known as data cleaning or data scrubbing, is the process of detecting and correcting (or removing) corrupt or inaccurate records from a dataset, table, or database. It involves identifying incomplete, incorrect, inaccurate, or irrelevant parts of the data and then replacing, modifying, or deleting this dirty data. Data cleansing is crucial for ensuring the quality and reliability of data used in various applications, including business intelligence, data analytics, and machine learning.
Importance of Data Cleansing
Data cleansing is essential for maintaining the integrity and accuracy of data. Poor data quality can lead to erroneous conclusions, faulty analyses, and misguided decision-making. Clean data ensures that analyses are based on reliable information, which is critical for achieving accurate results. Additionally, data cleansing helps in improving the efficiency of data processing and reducing the costs associated with data storage and management.
Common Data Quality Issues
Several common data quality issues necessitate data cleansing:
- **Duplicate Data**: Duplicate records can occur due to data entry errors, system migrations, or integration of multiple data sources.
- **Inconsistent Data**: Variations in data formats, units of measurement, or naming conventions can lead to inconsistencies.
- **Incomplete Data**: Missing values or incomplete records can hinder data analysis and decision-making.
- **Inaccurate Data**: Errors in data entry, outdated information, or incorrect data can result in inaccuracies.
- **Irrelevant Data**: Data that is no longer relevant or useful can clutter databases and reduce efficiency.
Data Cleansing Techniques
Data cleansing involves several techniques to address various data quality issues:
Deduplication
Deduplication is the process of identifying and removing duplicate records from a dataset. This can be achieved through various methods, such as exact matching, fuzzy matching, and record linkage. Exact matching involves comparing records based on identical values, while fuzzy matching uses algorithms to identify similar but not identical records.
Standardization
Standardization involves converting data into a consistent format. This includes standardizing date formats, units of measurement, and naming conventions. For example, dates can be standardized to a single format (e.g., YYYY-MM-DD), and units of measurement can be converted to a common unit (e.g., converting all weights to kilograms).
Imputation
Imputation is the process of filling in missing values in a dataset. Various methods can be used for imputation, including mean imputation, median imputation, and regression imputation. Mean imputation involves replacing missing values with the mean of the available data, while regression imputation uses regression models to predict missing values based on other variables.
Validation
Validation involves checking data for accuracy and consistency. This can be done through various validation rules, such as range checks, format checks, and consistency checks. Range checks ensure that data values fall within a specified range, format checks verify that data follows a specific format, and consistency checks ensure that related data fields are consistent with each other.
Enrichment
Data enrichment involves enhancing the quality of data by adding additional information from external sources. This can include appending missing information, correcting inaccuracies, or adding new attributes. For example, enriching customer data with demographic information can provide more insights for marketing analysis.
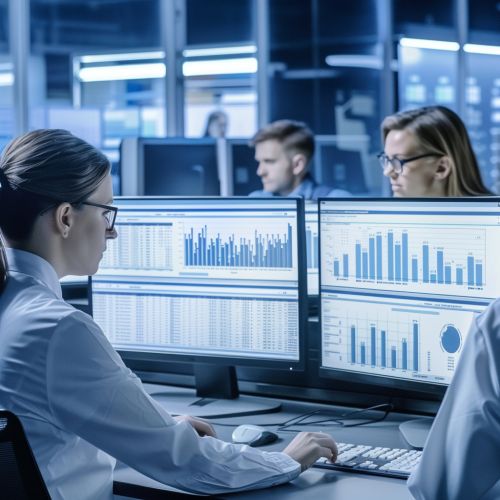
Tools and Technologies for Data Cleansing
Several tools and technologies are available for data cleansing, ranging from simple software applications to advanced data management platforms. Some popular data cleansing tools include:
- **OpenRefine**: An open-source tool for cleaning and transforming data.
- **Trifacta**: A data wrangling tool that provides an intuitive interface for data cleansing.
- **Talend**: An open-source data integration platform with data cleansing capabilities.
- **Informatica**: A data management platform that offers comprehensive data cleansing features.
- **Alteryx**: A data analytics platform that includes data cleansing tools.
Challenges in Data Cleansing
Data cleansing can be a complex and time-consuming process, with several challenges:
- **Volume of Data**: Large datasets can make data cleansing a daunting task, requiring significant computational resources and time.
- **Variety of Data Sources**: Integrating and cleansing data from multiple sources with different formats and structures can be challenging.
- **Dynamic Data**: Data that changes frequently can make it difficult to maintain data quality over time.
- **Subjectivity**: Determining what constitutes "clean" data can be subjective and may vary depending on the context and requirements.
Best Practices for Data Cleansing
To ensure effective data cleansing, several best practices should be followed:
- **Define Data Quality Standards**: Establish clear data quality standards and criteria for what constitutes clean data.
- **Automate Where Possible**: Use automated tools and scripts to streamline the data cleansing process and reduce manual effort.
- **Regularly Monitor Data Quality**: Continuously monitor data quality and perform regular data cleansing to maintain data integrity.
- **Document Processes**: Document data cleansing processes and procedures to ensure consistency and reproducibility.
- **Involve Stakeholders**: Engage relevant stakeholders, such as data owners and users, in the data cleansing process to ensure that data meets their needs and requirements.
Applications of Data Cleansing
Data cleansing is applicable in various domains and industries:
- **Business Intelligence**: Clean data is essential for accurate business intelligence and reporting.
- **Data Analytics**: High-quality data is crucial for reliable data analytics and insights.
- **Machine Learning**: Clean and accurate data is necessary for training effective machine learning models.
- **Healthcare**: Data cleansing is vital for maintaining accurate patient records and ensuring quality healthcare services.
- **Finance**: Clean data is critical for financial analysis, reporting, and compliance.
Future Trends in Data Cleansing
The field of data cleansing is continuously evolving, with several emerging trends:
- **Artificial Intelligence and Machine Learning**: AI and machine learning are being increasingly used to automate and enhance data cleansing processes.
- **Real-Time Data Cleansing**: The demand for real-time data processing is driving the development of real-time data cleansing solutions.
- **Data Governance**: The growing importance of data governance is leading to more stringent data quality standards and practices.
- **Cloud-Based Solutions**: Cloud-based data cleansing tools and platforms are becoming more popular due to their scalability and flexibility.
See Also
References
- [Reference 1]
- [Reference 2]
- [Reference 3]