Frequentist Statistics: Difference between revisions
No edit summary |
No edit summary |
||
Line 97: | Line 97: | ||
== Image == | == Image == | ||
[[Image:Detail-91969.jpg|thumb|center|A group of statisticians working on data analysis in a modern office environment.]] | [[Image:Detail-91969.jpg|thumb|center|A group of statisticians working on data analysis in a modern office environment.|class=only_on_mobile]] | ||
[[Image:Detail-91970.jpg|thumb|center|A group of statisticians working on data analysis in a modern office environment.|class=only_on_desktop]] | |||
== Categories == | == Categories == |
Latest revision as of 19:41, 18 June 2024
Introduction
Frequentist statistics, also known as classical statistics, is a branch of statistics that interprets probability as the long-run frequency of events. This approach to statistical inference is grounded in the idea that the probability of an event is the limit of its relative frequency in a large number of trials. Frequentist methods are widely used in scientific research, engineering, economics, and many other fields.
Historical Background
Frequentist statistics has its roots in the works of early statisticians such as Pierre-Simon Laplace, Carl Friedrich Gauss, and Ronald A. Fisher. Laplace's work on probability and Gauss's development of the normal distribution laid the foundation for frequentist methods. Fisher's contributions in the early 20th century, including the development of maximum likelihood estimation and the analysis of variance, further solidified the frequentist approach.
Key Concepts
Probability
In frequentist statistics, probability is defined as the long-run relative frequency of an event occurring in repeated trials. This interpretation contrasts with the Bayesian view, where probability is a measure of belief or certainty about an event.
Population and Sample
A population is the entire set of individuals or observations of interest, while a sample is a subset of the population selected for analysis. Frequentist methods often involve drawing random samples from a population to make inferences about population parameters.
Parameter Estimation
Parameter estimation is the process of using sample data to estimate the parameters of a population distribution. Common methods of parameter estimation in frequentist statistics include:
Hypothesis Testing
Hypothesis testing is a fundamental aspect of frequentist statistics. It involves formulating a null hypothesis (H0) and an alternative hypothesis (H1), and using sample data to determine whether there is enough evidence to reject the null hypothesis. Key concepts in hypothesis testing include:
Confidence Intervals
A confidence interval is a range of values, derived from sample data, that is likely to contain the true value of a population parameter. Confidence intervals provide a measure of uncertainty around parameter estimates and are typically expressed at a specified confidence level (e.g., 95%).
Frequentist Methods
Maximum Likelihood Estimation (MLE)
Maximum likelihood estimation is a method for estimating the parameters of a statistical model. It involves finding the parameter values that maximize the likelihood function, which measures the probability of observing the sample data given the parameters. MLE is widely used due to its desirable properties, such as consistency and asymptotic normality.
Hypothesis Testing
Hypothesis testing in frequentist statistics involves several steps:
1. Formulate the null and alternative hypotheses. 2. Choose a significance level (α). 3. Select an appropriate test statistic. 4. Determine the critical value(s) or p-value. 5. Make a decision to reject or fail to reject the null hypothesis.
Common hypothesis tests include:
Confidence Intervals
Constructing a confidence interval involves:
1. Selecting a confidence level (e.g., 95%). 2. Calculating the sample statistic (e.g., sample mean). 3. Determining the standard error of the statistic. 4. Using the appropriate distribution to find the critical value. 5. Constructing the interval using the sample statistic, standard error, and critical value.
Applications
Frequentist methods are applied in various fields, including:
- Clinical Trials: Evaluating the efficacy and safety of new treatments.
- Quality Control: Monitoring and improving manufacturing processes.
- Econometrics: Analyzing economic data and testing economic theories.
- Psychometrics: Measuring psychological traits and abilities.
Criticisms and Alternatives
Frequentist statistics has been criticized for its reliance on long-run frequencies and its interpretation of probability. Critics argue that this approach can be limiting in situations where repeated trials are not feasible or where subjective beliefs are important. Alternatives to frequentist statistics include:
- Bayesian Statistics: Interprets probability as a measure of belief and incorporates prior information.
- Likelihoodist Statistics: Focuses on the likelihood function without relying on long-run frequencies.
See Also
- Bayesian statistics
- Likelihoodist statistics
- Statistical inference
- Probability theory
- Sampling (statistics)
Image
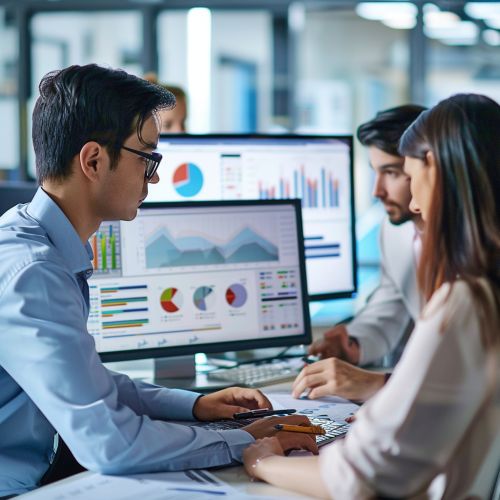
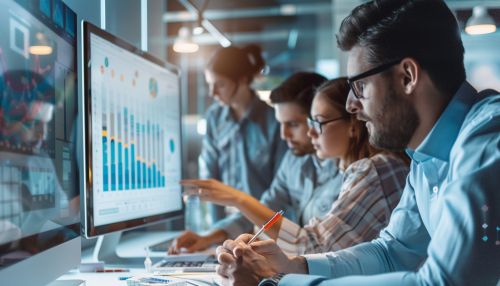