Kruskal-Wallis test: Difference between revisions
(Created page with "== Introduction == The Kruskal-Wallis test is a non-parametric statistical method used to determine if there are statistically significant differences between the medians of three or more independent groups. Named after William Kruskal and W. Allen Wallis, this test is an extension of the Mann-Whitney U test, which is used for comparing two groups. The Kruskal-Wallis test is particularly useful when the assumptions of the Analysis of Variance (ANOVA) are n...") |
No edit summary |
||
Line 37: | Line 37: | ||
A significant result indicates that at least one group median is different from the others. However, it does not specify which groups are different. Post-hoc tests, such as the [[Dunn's test]], are often used to identify specific group differences. | A significant result indicates that at least one group median is different from the others. However, it does not specify which groups are different. Post-hoc tests, such as the [[Dunn's test]], are often used to identify specific group differences. | ||
[[Image:Detail-98543.jpg|thumb|center|A diverse group of people engaging in a collaborative discussion around a table, with papers and a laptop visible.|class=only_on_mobile]] | |||
[[Image:Detail-98544.jpg|thumb|center|A diverse group of people engaging in a collaborative discussion around a table, with papers and a laptop visible.|class=only_on_desktop]] | |||
== Applications == | == Applications == |
Latest revision as of 01:02, 19 October 2024
Introduction
The Kruskal-Wallis test is a non-parametric statistical method used to determine if there are statistically significant differences between the medians of three or more independent groups. Named after William Kruskal and W. Allen Wallis, this test is an extension of the Mann-Whitney U test, which is used for comparing two groups. The Kruskal-Wallis test is particularly useful when the assumptions of the Analysis of Variance (ANOVA) are not met, specifically when the data do not follow a normal distribution or when variances are unequal.
Background and Development
The Kruskal-Wallis test was developed in the early 1950s as a robust alternative to parametric methods like ANOVA. The need for such a test arose from the limitations of parametric tests, which require data to meet certain assumptions such as normality and homogeneity of variance. The Kruskal-Wallis test, being non-parametric, does not require these assumptions, making it suitable for ordinal data or continuous data that do not meet parametric assumptions.
Methodology
Assumptions
While the Kruskal-Wallis test is less restrictive than ANOVA, it still has some assumptions:
1. **Independence**: The samples must be independent of each other. 2. **Ordinal or Continuous Data**: The data should be at least ordinal. 3. **Similar Shape of Distributions**: The distributions of the groups should have a similar shape.
Test Procedure
The procedure for conducting a Kruskal-Wallis test involves the following steps:
1. **Rank All Data**: Combine all data from the groups and rank them from smallest to largest. 2. **Calculate Rank Sums**: Compute the sum of ranks for each group. 3. **Compute the Test Statistic**: Use the rank sums to calculate the Kruskal-Wallis statistic, \( H \), which is given by:
\[ H = \frac{12}{N(N+1)} \sum \frac{R_i^2}{n_i} - 3(N+1) \]
where \( N \) is the total number of observations, \( R_i \) is the sum of ranks for group \( i \), and \( n_i \) is the number of observations in group \( i \).
4. **Determine Significance**: Compare the computed \( H \) value against the chi-squared distribution with \( k-1 \) degrees of freedom, where \( k \) is the number of groups.
Interpretation
A significant result indicates that at least one group median is different from the others. However, it does not specify which groups are different. Post-hoc tests, such as the Dunn's test, are often used to identify specific group differences.
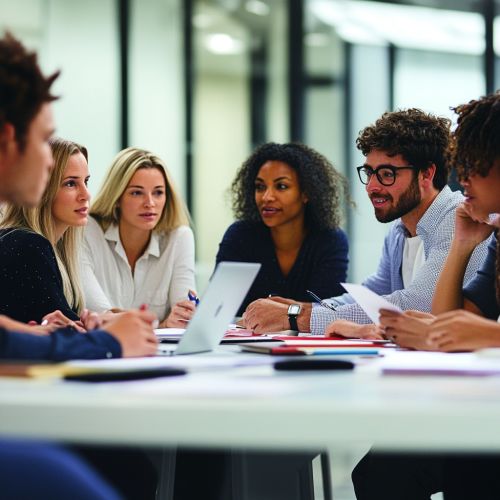
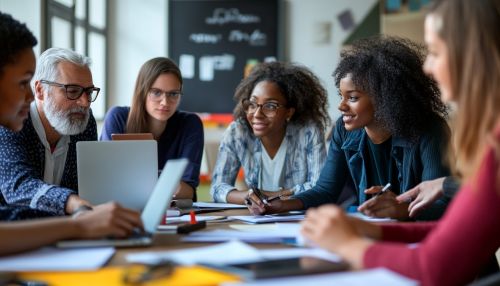
Applications
The Kruskal-Wallis test is widely used in various fields, including:
- **Biology**: To compare growth rates of different species under various conditions. - **Medicine**: To evaluate the effectiveness of different treatments across patient groups. - **Social Sciences**: To assess differences in survey responses across demographic groups.
Advantages and Limitations
Advantages
- **Non-parametric Nature**: Does not require normal distribution. - **Robustness**: Handles outliers and skewed data effectively. - **Versatility**: Applicable to ordinal data.
Limitations
- **Less Powerful**: Generally less powerful than parametric tests when assumptions are met. - **No Pairwise Comparisons**: Does not identify which groups differ. - **Assumption of Similar Distribution Shapes**: Requires similar distribution shapes across groups.