Ontological Engineering: Difference between revisions
(Created page with "== Introduction == Ontological engineering is a subfield of artificial intelligence (AI) and knowledge management that focuses on the design and creation of ontologies. Ontologies are formal representations of a set of concepts within a domain and the relationships between those concepts. This field is critical for enabling machines to understand and process complex information in a way that is meaningful and useful for various applications, including semantic web, natu...") |
No edit summary |
||
Line 91: | Line 91: | ||
The future of ontological engineering lies in addressing these challenges and expanding the applicability of ontologies. Advances in machine learning and AI may offer new ways to automate ontology development and maintenance. Additionally, the integration of ontologies with emerging technologies like the Internet of Things (IoT) and blockchain holds promise for creating more intelligent and interconnected systems. | The future of ontological engineering lies in addressing these challenges and expanding the applicability of ontologies. Advances in machine learning and AI may offer new ways to automate ontology development and maintenance. Additionally, the integration of ontologies with emerging technologies like the Internet of Things (IoT) and blockchain holds promise for creating more intelligent and interconnected systems. | ||
[[Image:Detail-98103.jpg|thumb|center|A detailed diagram of an ontology representing various interconnected concepts within a specific domain.|class=only_on_mobile]] | |||
[[Image:Detail-98104.jpg|thumb|center|A detailed diagram of an ontology representing various interconnected concepts within a specific domain.|class=only_on_desktop]] | |||
== See Also == | == See Also == |
Latest revision as of 01:15, 25 September 2024
Introduction
Ontological engineering is a subfield of artificial intelligence (AI) and knowledge management that focuses on the design and creation of ontologies. Ontologies are formal representations of a set of concepts within a domain and the relationships between those concepts. This field is critical for enabling machines to understand and process complex information in a way that is meaningful and useful for various applications, including semantic web, natural language processing, and information retrieval.
Historical Background
The concept of ontological engineering emerged from the broader field of knowledge representation and reasoning in AI. Early work in this area can be traced back to the 1970s and 1980s, with the development of frame-based systems and semantic networks. These early systems laid the groundwork for more sophisticated ontological frameworks that would come later.
In the 1990s, the advent of the World Wide Web and the need for more advanced information retrieval systems spurred significant interest in ontological engineering. Researchers began to develop standardized languages and tools for creating and managing ontologies, such as the Resource Description Framework (RDF) and the Web Ontology Language (OWL).
Core Concepts
Ontologies
An ontology is a formal, explicit specification of a shared conceptualization. It consists of a set of concepts, also known as classes or types, and the relationships between them. Ontologies are used to model domains of knowledge and are essential for enabling interoperability between different systems.
Classes and Instances
In ontological engineering, a class represents a category of objects or concepts within a domain. For example, in a medical ontology, "Disease" might be a class. Instances are specific examples of these classes, such as "Influenza" being an instance of the "Disease" class.
Properties and Relationships
Properties are attributes or characteristics of classes and instances. Relationships, on the other hand, define how classes and instances are connected to one another. For example, in a geographical ontology, the relationship "isLocatedIn" might connect the class "City" to the class "Country".
Axioms and Rules
Axioms are statements that are always true within the context of the ontology. They are used to define constraints and infer new information. Rules, often expressed in languages like SWRL (Semantic Web Rule Language), provide a way to derive new knowledge from existing facts.
Ontology Development Process
The process of developing an ontology typically involves several stages:
Requirements Analysis
This initial stage involves identifying the scope and purpose of the ontology. Stakeholders and domain experts collaborate to determine what concepts and relationships need to be represented.
Conceptualization
During this phase, the identified concepts and relationships are organized into a conceptual model. This model serves as a blueprint for the ontology and helps ensure that all relevant aspects of the domain are covered.
Formalization
In the formalization stage, the conceptual model is translated into a formal representation using an ontology language such as OWL or RDF. This involves defining classes, properties, relationships, and axioms.
Implementation
The formalized ontology is then implemented using ontology development tools like Protégé or OntoEdit. This stage may also involve integrating the ontology with other systems and data sources.
Evaluation and Iteration
Once the ontology is implemented, it must be evaluated to ensure it meets the requirements and accurately represents the domain. This may involve testing the ontology with real-world data and making iterative improvements based on feedback.
Applications of Ontological Engineering
Ontological engineering has a wide range of applications across various fields:
Semantic Web
Ontologies are a cornerstone of the Semantic Web, enabling machines to understand and process web content in a meaningful way. They facilitate data integration, interoperability, and more advanced search capabilities.
Natural Language Processing
In NLP, ontologies are used to enhance the understanding of text by providing a structured representation of knowledge. This can improve tasks such as information extraction, sentiment analysis, and machine translation.
Information Retrieval
Ontologies improve information retrieval systems by providing a more nuanced understanding of user queries and the relationships between different pieces of information. This leads to more accurate and relevant search results.
Healthcare and Bioinformatics
In healthcare, ontologies are used to model complex medical knowledge, enabling better data sharing and decision support. For example, the Gene Ontology provides a structured representation of gene and protein functions, facilitating research in bioinformatics.
Challenges and Future Directions
Despite its many benefits, ontological engineering faces several challenges:
Scalability
Creating and managing large-scale ontologies can be resource-intensive and complex. Ensuring that ontologies remain up-to-date and relevant as domains evolve is an ongoing challenge.
Interoperability
While ontologies aim to facilitate interoperability, achieving seamless integration between different ontologies and systems remains difficult. Standardization efforts are ongoing, but more work is needed to achieve true interoperability.
Usability
Developing ontologies requires specialized knowledge and skills, which can be a barrier to adoption. Improving the usability of ontology development tools and making the process more accessible to non-experts is a key area of focus.
Future Directions
The future of ontological engineering lies in addressing these challenges and expanding the applicability of ontologies. Advances in machine learning and AI may offer new ways to automate ontology development and maintenance. Additionally, the integration of ontologies with emerging technologies like the Internet of Things (IoT) and blockchain holds promise for creating more intelligent and interconnected systems.
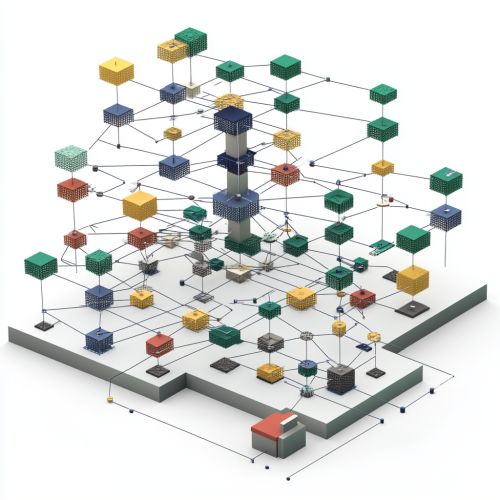
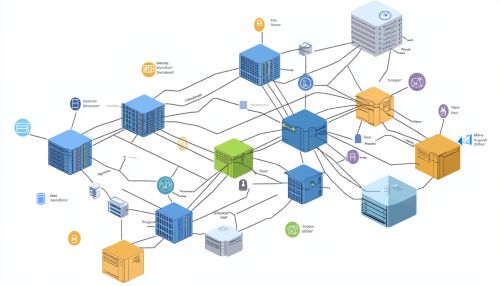
See Also
- Knowledge Representation
- Semantic Web
- Natural Language Processing
- Information Retrieval
- Gene Ontology