P-value: Difference between revisions
(Created page with "== Introduction == The p-value, or probability value, is a fundamental concept in statistical hypothesis testing. It is used to quantify the evidence against a null hypothesis. The p-value represents the probability of obtaining test results at least as extreme as the observed results, assuming that the null hypothesis is true. In essence, it helps researchers determine whether their findings are statistically significant. == Calculation of P-value == The calculatio...") |
No edit summary |
||
Line 53: | Line 53: | ||
The p-value is a crucial tool in statistical hypothesis testing, providing a measure of the evidence against the null hypothesis. While it has its limitations and potential for misuse, it remains a fundamental component of statistical analysis. By understanding its calculation, interpretation, and context, researchers can make more informed decisions about their data. | The p-value is a crucial tool in statistical hypothesis testing, providing a measure of the evidence against the null hypothesis. While it has its limitations and potential for misuse, it remains a fundamental component of statistical analysis. By understanding its calculation, interpretation, and context, researchers can make more informed decisions about their data. | ||
[[Image:Detail-92931.jpg|thumb|center|A scientist analyzing data on a computer in a laboratory setting.|class=only_on_mobile]] | |||
[[Image:Detail-92932.jpg|thumb|center|A scientist analyzing data on a computer in a laboratory setting.|class=only_on_desktop]] | |||
== See Also == | == See Also == |
Latest revision as of 20:01, 21 June 2024
Introduction
The p-value, or probability value, is a fundamental concept in statistical hypothesis testing. It is used to quantify the evidence against a null hypothesis. The p-value represents the probability of obtaining test results at least as extreme as the observed results, assuming that the null hypothesis is true. In essence, it helps researchers determine whether their findings are statistically significant.
Calculation of P-value
The calculation of the p-value depends on the statistical test being used. Common tests include the t-test, chi-squared test, and ANOVA. Each test has its own method for calculating the p-value, but they all follow a similar process:
1. **Formulate Hypotheses**: Define the null hypothesis (H0) and the alternative hypothesis (H1). 2. **Select Significance Level**: Choose a significance level (α), commonly set at 0.05. 3. **Compute Test Statistic**: Calculate the test statistic based on the sample data. 4. **Determine P-value**: Use the test statistic to find the p-value from the appropriate statistical distribution.
Interpretation of P-value
The p-value is interpreted in the context of the chosen significance level (α). If the p-value is less than or equal to α, the null hypothesis is rejected, indicating that the observed data is unlikely under the null hypothesis. Conversely, if the p-value is greater than α, the null hypothesis is not rejected.
A common misconception is that a p-value indicates the probability that the null hypothesis is true. Instead, it measures the probability of observing the data given that the null hypothesis is true.
P-value Thresholds
The threshold for significance, often denoted as α, is a critical value that determines whether the p-value indicates statistical significance. Common thresholds include:
- **α = 0.05**: Standard threshold for significance.
- **α = 0.01**: More stringent threshold, reducing the likelihood of Type I errors.
- **α = 0.10**: Less stringent threshold, increasing the likelihood of detecting an effect.
P-value in Context
P-values are often used in conjunction with other statistical measures, such as confidence intervals and effect sizes, to provide a more comprehensive understanding of the data. While a small p-value indicates statistical significance, it does not provide information about the magnitude or practical significance of the effect.
Criticisms and Misinterpretations
P-values have been subject to various criticisms and misinterpretations. Some common issues include:
- **P-hacking**: Manipulating data or analysis methods to achieve a desirable p-value.
- **Overemphasis on P-values**: Relying solely on p-values without considering other statistical measures.
- **Misinterpretation**: Incorrectly interpreting p-values as the probability that the null hypothesis is true.
Alternatives to P-value
Several alternatives to p-values have been proposed to address their limitations. These include:
- **Bayesian Methods**: Incorporate prior information and provide a probability distribution for the parameters of interest.
- **Effect Sizes**: Quantify the magnitude of an effect, providing more context than a p-value alone.
- **Confidence Intervals**: Provide a range of values within which the true parameter is likely to lie.
Historical Context
The concept of the p-value was introduced by Ronald A. Fisher in the early 20th century. Fisher's work laid the foundation for modern statistical hypothesis testing and the widespread use of p-values in scientific research.
Practical Applications
P-values are used across various fields, including:
- **Medicine**: Evaluating the efficacy of treatments and interventions.
- **Psychology**: Testing hypotheses about human behavior and cognitive processes.
- **Economics**: Analyzing economic data and testing economic theories.
- **Environmental Science**: Assessing the impact of environmental changes and interventions.
Conclusion
The p-value is a crucial tool in statistical hypothesis testing, providing a measure of the evidence against the null hypothesis. While it has its limitations and potential for misuse, it remains a fundamental component of statistical analysis. By understanding its calculation, interpretation, and context, researchers can make more informed decisions about their data.
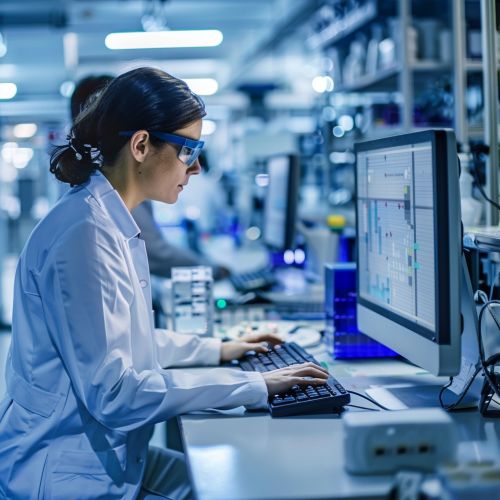
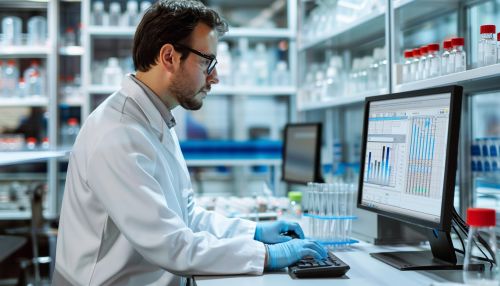