Molecular Modelling: Difference between revisions
(Created page with "== Introduction == Molecular modelling is a collective term that refers to theoretical methods and computational techniques used to model or mimic the behavior of molecules. The techniques are used in the fields of computational chemistry, drug design, computational biology, and materials science to study molecular systems ranging from small chemical systems to large biological molecules and material assemblies. Molecular modelling encompasses all methods, theoretical an...") |
No edit summary |
||
Line 26: | Line 26: | ||
Molecular dynamics (MD) simulations involve solving Newton's equations of motion for a system of interacting particles. MD simulations provide insights into the time-dependent behavior of molecular systems, such as conformational changes, diffusion, and protein-ligand interactions. Techniques such as the Verlet algorithm and the leapfrog algorithm are commonly used to integrate the equations of motion. | Molecular dynamics (MD) simulations involve solving Newton's equations of motion for a system of interacting particles. MD simulations provide insights into the time-dependent behavior of molecular systems, such as conformational changes, diffusion, and protein-ligand interactions. Techniques such as the Verlet algorithm and the leapfrog algorithm are commonly used to integrate the equations of motion. | ||
[[Image:Detail-93123.jpg|thumb|center|Visualization of a protein-ligand complex. The protein is shown in ribbon representation, and the ligand is shown in stick representation.|class=only_on_mobile]] | |||
[[Image:Detail-93124.jpg|thumb|center|Visualization of a protein-ligand complex. The protein is shown in ribbon representation, and the ligand is shown in stick representation.|class=only_on_desktop]] | |||
== Applications == | == Applications == |
Latest revision as of 23:42, 21 June 2024
Introduction
Molecular modelling is a collective term that refers to theoretical methods and computational techniques used to model or mimic the behavior of molecules. The techniques are used in the fields of computational chemistry, drug design, computational biology, and materials science to study molecular systems ranging from small chemical systems to large biological molecules and material assemblies. Molecular modelling encompasses all methods, theoretical and computational, used to model or mimic the behavior of molecules.
Historical Background
The origins of molecular modelling can be traced back to the early 20th century with the development of quantum mechanics and the Schrödinger equation. The advent of computers in the mid-20th century allowed for the practical application of these theoretical concepts to real-world molecular systems. Early pioneers such as Linus Pauling and Robert Mulliken laid the groundwork for the field, which has since evolved with advances in computational power and algorithm development.
Fundamental Concepts
Molecular modelling relies heavily on the principles of quantum mechanics and classical mechanics. The Schrödinger equation is central to quantum mechanical models, while classical mechanics often employs force fields to describe the interactions between atoms and molecules.
Quantum Mechanics
Quantum mechanics provides a fundamental framework for understanding the behavior of electrons in molecules. The Schrödinger equation describes how the quantum state of a physical system changes over time. Solutions to the Schrödinger equation provide information about molecular orbitals, electron densities, and potential energy surfaces.
Classical Mechanics
In classical mechanics, molecular systems are often modeled using force fields, which are mathematical functions that describe the potential energy of a system as a function of the positions of its atoms. Force fields include terms for bond stretching, angle bending, torsional angles, and non-bonded interactions such as van der Waals forces and electrostatic interactions.
Computational Techniques
Molecular modelling employs a variety of computational techniques, each with its own strengths and limitations. These techniques can be broadly classified into quantum mechanical methods, molecular mechanics, and molecular dynamics.
Quantum Mechanical Methods
Quantum mechanical methods include ab initio and semi-empirical methods. Ab initio methods, such as Hartree-Fock and post-Hartree-Fock methods, do not rely on empirical data and are based solely on quantum mechanical principles. Semi-empirical methods, such as the AM1 and PM3 methods, incorporate empirical data to simplify calculations and reduce computational cost.
Molecular Mechanics
Molecular mechanics uses force fields to model molecular systems. Common force fields include AMBER, CHARMM, and OPLS. These force fields are parameterized based on experimental data and high-level quantum mechanical calculations. Molecular mechanics is particularly useful for large systems where quantum mechanical methods would be computationally prohibitive.
Molecular Dynamics
Molecular dynamics (MD) simulations involve solving Newton's equations of motion for a system of interacting particles. MD simulations provide insights into the time-dependent behavior of molecular systems, such as conformational changes, diffusion, and protein-ligand interactions. Techniques such as the Verlet algorithm and the leapfrog algorithm are commonly used to integrate the equations of motion.
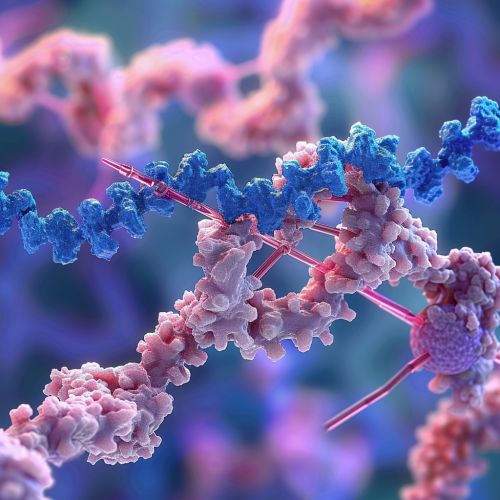
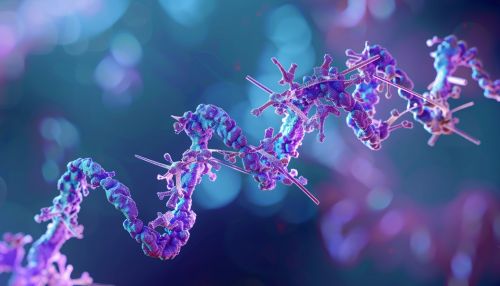
Applications
Molecular modelling has a wide range of applications in various scientific disciplines.
Drug Design
In drug design, molecular modelling is used to predict the binding affinity of potential drug candidates to their target proteins. Techniques such as molecular docking and quantitative structure-activity relationship (QSAR) models are commonly employed. Molecular docking involves predicting the preferred orientation of a small molecule when bound to a protein, while QSAR models relate chemical structure to biological activity.
Materials Science
In materials science, molecular modelling is used to study the properties of materials at the atomic and molecular levels. This includes the design of new materials with specific properties, such as increased strength, conductivity, or reactivity. Techniques such as density functional theory (DFT) and molecular dynamics are commonly used.
Computational Biology
In computational biology, molecular modelling is used to study the structure and function of biological macromolecules, such as proteins, nucleic acids, and membranes. Techniques such as homology modelling, molecular dynamics, and free energy calculations are employed to understand biological processes at the molecular level.
Challenges and Limitations
Despite its many successes, molecular modelling faces several challenges and limitations.
Computational Cost
High-level quantum mechanical methods are computationally expensive and often impractical for large systems. Even with advances in computational power, the size and complexity of biological systems pose significant challenges.
Accuracy of Force Fields
The accuracy of molecular mechanics simulations depends on the quality of the force fields used. Force fields are parameterized based on empirical data and high-level quantum mechanical calculations, but they may not accurately capture all the nuances of molecular interactions.
Sampling Issues
Molecular dynamics simulations require extensive sampling to obtain statistically meaningful results. This can be particularly challenging for systems with high energy barriers or slow conformational changes.
Future Directions
The field of molecular modelling is continuously evolving, with ongoing developments in computational techniques and hardware.
Machine Learning
Machine learning techniques are increasingly being integrated into molecular modelling workflows. These techniques can be used to develop more accurate force fields, predict molecular properties, and accelerate the discovery of new materials and drugs.
Quantum Computing
Quantum computing holds the potential to revolutionize molecular modelling by providing the computational power needed to solve complex quantum mechanical problems. While still in its early stages, quantum computing could eventually enable accurate simulations of large molecular systems.
Enhanced Sampling Techniques
Enhanced sampling techniques, such as metadynamics and replica exchange molecular dynamics, are being developed to address sampling issues in molecular dynamics simulations. These techniques aim to improve the efficiency of sampling and provide more accurate insights into molecular behavior.