Bayesian Hierarchical Model: Difference between revisions
(Created page with "== Introduction == A Bayesian hierarchical model is a statistical model that involves multiple levels of random variables, with Bayesian inference used to estimate the parameters of the model. These models are particularly useful for analyzing data that has a natural hierarchical structure, such as data collected from multiple groups or clusters. Bayesian hierarchical models allow for the incorporation of prior knowledge and the sharing of information across different l...") |
No edit summary |
||
Line 69: | Line 69: | ||
* [[Gibbs Sampling]] | * [[Gibbs Sampling]] | ||
[[Image:Detail-91757.jpg|thumb|center|Illustration of a Bayesian hierarchical model with multiple levels of random variables.|class=only_on_mobile]] | |||
[[Image:Detail-91758.jpg|thumb|center|Illustration of a Bayesian hierarchical model with multiple levels of random variables.|class=only_on_desktop]] | |||
== References == | == References == |
Latest revision as of 19:21, 19 June 2024
Introduction
A Bayesian hierarchical model is a statistical model that involves multiple levels of random variables, with Bayesian inference used to estimate the parameters of the model. These models are particularly useful for analyzing data that has a natural hierarchical structure, such as data collected from multiple groups or clusters. Bayesian hierarchical models allow for the incorporation of prior knowledge and the sharing of information across different levels of the hierarchy, leading to more robust and reliable inferences.
Structure of Bayesian Hierarchical Models
Bayesian hierarchical models consist of multiple levels, each representing a different layer of the hierarchy. The basic structure includes:
1. **Data Level (Observation Level)**: This is the level at which the observed data is modeled. The data is assumed to be generated from a probability distribution that depends on certain parameters. 2. **Parameter Level (Group Level)**: This level models the parameters that govern the distribution of the data. These parameters are treated as random variables with their own probability distributions. 3. **Hyperparameter Level (Population Level)**: This level models the hyperparameters, which are the parameters of the prior distributions of the group-level parameters. Hyperparameters are also treated as random variables with their own prior distributions.
The hierarchical structure allows for the modeling of complex dependencies and the sharing of information across different levels of the hierarchy.
Bayesian Inference in Hierarchical Models
Bayesian inference involves updating the probability distributions of the parameters based on the observed data. In the context of hierarchical models, this process involves several steps:
1. **Specification of Prior Distributions**: Prior distributions are specified for the parameters at each level of the hierarchy. These priors represent the initial beliefs about the parameters before observing the data. 2. **Likelihood Function**: The likelihood function is specified, which describes the probability of the observed data given the parameters. 3. **Posterior Distribution**: The posterior distribution is computed using Bayes' theorem. This distribution represents the updated beliefs about the parameters after observing the data. The posterior distribution is proportional to the product of the prior distribution and the likelihood function.
Applications of Bayesian Hierarchical Models
Bayesian hierarchical models have a wide range of applications in various fields, including:
Medicine
In medical research, hierarchical models are used to analyze data from multiple clinical trials or studies. For example, a meta-analysis can be conducted using a hierarchical model to combine results from different studies and estimate the overall effect size.
Education
In educational research, hierarchical models are used to analyze data from students nested within schools. These models can account for the variability between schools and provide more accurate estimates of student performance.
Marketing
In marketing research, hierarchical models are used to analyze data from consumers nested within different market segments. These models can help identify segment-specific preferences and improve targeted marketing strategies.
Environmental Science
In environmental science, hierarchical models are used to analyze spatial and temporal data. For example, a hierarchical model can be used to study the distribution of a species across different regions and time periods.
Advantages of Bayesian Hierarchical Models
Bayesian hierarchical models offer several advantages over traditional statistical models:
1. **Incorporation of Prior Knowledge**: Bayesian models allow for the incorporation of prior knowledge through the specification of prior distributions. This can lead to more accurate and robust inferences, especially in cases where data is sparse or noisy. 2. **Pooling of Information**: Hierarchical models allow for the pooling of information across different levels of the hierarchy. This can lead to more precise estimates of parameters and improved predictions. 3. **Flexibility**: Bayesian hierarchical models are highly flexible and can be adapted to a wide range of data structures and applications. They can accommodate complex dependencies and interactions between variables.
Challenges and Limitations
Despite their advantages, Bayesian hierarchical models also present several challenges and limitations:
1. **Computational Complexity**: Bayesian inference in hierarchical models can be computationally intensive, especially for large datasets or complex models. Advanced computational techniques, such as Markov Chain Monte Carlo (MCMC) methods, are often required to approximate the posterior distributions. 2. **Model Specification**: The specification of prior distributions and the likelihood function can be challenging, especially in the absence of strong prior knowledge. The choice of priors can have a significant impact on the results, and sensitivity analyses are often necessary to assess the robustness of the inferences. 3. **Interpretation**: The interpretation of the results from Bayesian hierarchical models can be complex, especially for non-statisticians. The hierarchical structure and the use of prior distributions can complicate the interpretation of the parameter estimates and their uncertainty.
Conclusion
Bayesian hierarchical models are powerful tools for analyzing data with a natural hierarchical structure. They allow for the incorporation of prior knowledge, the pooling of information across different levels of the hierarchy, and the modeling of complex dependencies. Despite their computational complexity and challenges in model specification, Bayesian hierarchical models offer significant advantages in terms of flexibility and robustness. They have a wide range of applications in various fields, including medicine, education, marketing, and environmental science.
See Also
- Bayesian Inference
- Markov Chain Monte Carlo
- Meta-Analysis
- Hierarchical Linear Modeling
- Gibbs Sampling
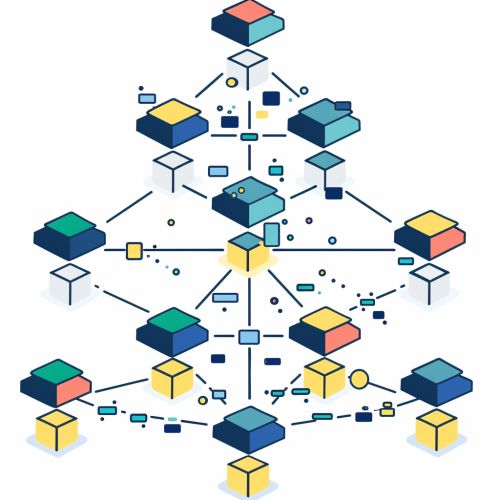
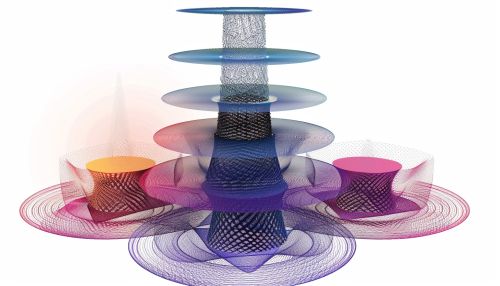